Intralogistics Automation and the Factories of the Future
Intralogistics 5.0 leverages automated material handling, Internet of Things (IoT), data analytics, and Artificial Intelligence (AI) to create smart, interconnected, data-driven production environments and empower human workers.
Request A Meeting
Please fill out your details below. Our team will reach out to you by email to schedule a date and time.
Autonomous Mobile Robots Powering Industry 5.0
Industry 5.0 is a new phase of industrialization that builds upon the fourth industrial revolution (Industry 4.0) by adding a people-centric approach. It is characterized by the extensive use of automation, data exchange, and smart manufacturing technologies in a manner that fosters the well-being of the workers. It bridges the gap of the unfulfilled promise of full automation from Industry 4.0 in processes where a human in the loop is still needed, increasing the competitiveness of the industry and helping attract the best talents.
The ultimate goal is to create human-centric, efficient, flexible, and responsive production environments that can quickly adapt to changing market demands.
To achieve this goal, this next generation of manufacturing environments leverages the latest available technologies to the entire production process. One of the key operational aspects of manufacturing is intralogistics -understood as the management, control, and optimization of internal material and information flows.
Intralogistics 5.0 leverages automated material handling, Internet of Things (IoT), data analytics, and Artificial Intelligence (AI) to create smart, interconnected, data-driven production environments and empower human workers.
This intralogistics evolution is not only adding efficiency to industrial processes but is also driving a shift in manufacturing, from large, rigid systems into modular, flexible, and robot-supported self-optimizing ones.
Optimizing Material Handling with Visual AI-Enabled Robotic Vehicles
Autonomous Mobile Robots (AMRs), equipped with Visual AI and orchestrated through a Fleet Management System (FMS) are at the forefront of this transformation. The shape and function of these AMRs range from autonomous forklifts, shuttle systems, tugger trains, and stackers to platform or mouse robots, goods-to-person, and pick-assist robots. Their material transport activities are ideally tracked directly by the Enterprise Resource Planning (ERP) system of the factory.
AMRs are designed to navigate with agility and accuracy through complex factory environments using a combination of sensors, cameras, and real-time data processing. The integration of Visual AI enables them to perceive their surroundings, identify objects and obstacles, make informed decisions, and share information in real-time, adding a number of benefits at each step of the process:
- Enhanced Goods-In: Vehicles equipped with Visual AI contribute to this stage by autonomously transporting goods from receiving areas to designated storage locations reducing manual work and extending operation hours. Additionally, the visual input ensures accurate identification and categorization of incoming goods, feeding the ERP, and laying the foundation for precise inventory management.
- Optimized Storage and Retrieval: Visual AI allows robotic vehicles to analyze available storage space and stack goods efficiently, optimizing storage capacity, ensuring timely retrieval of items, and providing real-time inventory updates.
- Streamlined Production Supply: Visual AI makes autonomous intralogistics vehicles inherently flexible, thus they can optimize routes dynamically, ensuring a continuous and streamlined supply of materials to production lines.
- Accurate Order Picking: Visual AI technology supports workers in order picking, significantly reducing error rates and ensuring accurate order fulfillment.
- Efficient Dispatch and Goods-Out: Visual AI enables robotic logistics vehicles to perform order verification and optimize load onto transportation vehicles, adding efficiency to the preparation and organization of finished goods for delivery to customers or distribution centers.
Flexible Manufacturing
Besides the optimization across the material handling process, and on account of their inherent reconfigurability, Visual AI AMRs can flexibly support diverse production scenarios, such as batch, mass, or customized production, and different manufacturing layouts, from linear or modular to cellular.
Serving as flexible links between individual production stages, these robots are also fundamental in the transition from traditional rigid conveyor-belt-based manufacturing to flexible-cell manufacturing, where production units can be reconfigured and rearranged without downtime.
This flexible movement and connection of production cells, create dynamic and modular production environments that can adapt to different product variants and volumes, to respond to changing customer demands and market conditions.
Challenges on the Path Towards the Factories of the Future
Visual AI allows operating this Factory of the Future today. While the technology is already here, its adaption and implementation are only starting to pick up speed. The transition to Intralogistics 5.0 and new levels of automation is mostly gradual and incremental as it requires interoperability, scalability, and human-robot collaboration.
Interoperability
Many manufacturing facilities and warehouses are currently operating with mixed fleets that consist of both manned and unmanned material-handling vehicles, and an initial and crucial challenge in the path toward Intralogistics 5.0 is to integrate both types of shop floor traffic into an all-encompassing FMS and ERP.
At this stage, equipping manned vehicles -like forklifts, tuggers, or stackers- with Visual AI-enabled Real Time Location System (RTLS) to track their location and feed the system with the visual data they capture would enable an FMS to orchestrate floor traffic holistically.
Adding observability to manned traffic and integrating it into an FMS cancels out typical shortcomings of mixed fleets such as human drivers colliding with AMRs, inefficient manned routing unobservable by the FMS, or inventory compromised by human errors.

The Visual AI-enabled RTLS adds value in manufacturing and warehousing unlocking completely new opportunities in material handling applications, where some operations will continue to be performed using manned vehicles for the next 20+ years.
To make the benefits of holistically managed mixed fleets broadly accessible to the market, Sevensense Robotics is developing a Visual AI-enabled RTLS, which is already available to early adopters.
Scalability
In many cases, as part of their automation journey, manufacturers opt for initially commissioning AMRs at a small scale in a dedicated sector of their facilities. However, expanding the AMRs’ operating space from a wing of a plant or to the entire factory has traditionally been time-consuming.
Until now, it implied a complex and disruptive endeavor of stopping operations and dedicating specialized professionals to the map expansion. including running a vehicle in manual mode to scan the new areas of the facility where robots will operate, and then manually editing and merging the new maps.

To solve this scalability blocker, Sevensense has developed a solution that makes the map expansion seamless. Combining continuous map updates -also known as Lifelong Visual SLAM- with swarm intelligence, robots equipped with this technology can automatically map the new sections of the environment and share the extended map with the rest of the fleet. All they need to start their exploration is an anchor point in the existing map and the expansion is computed on-the-fly.
By automatically incorporating relevant changes in the environment, Sevensense’s Lifelong Visual SLAM also offers the key benefit of lifelong robust, high precision localization <5mm (<0.2in) without markers, reflectors, or beacons, allowing the robots to operate in dynamic, ever-changing environments.
Human-Robot Collaboration
An increasing number of companies are incorporating AMRs into their operations. However, the lack of seamless human-machine collaboration solutions poses the need for dedicated robotic operations zones or the introduction of robotic shifts, restricting their flexibility and efficiency while also limiting the ROI.
Visual AI AMRs have the built-in capability of understanding the context of the environment, distinguishing between unobstructed paths, obstacles -and types of obstacles-, and adapting their behavior accordingly in real-time.
When the robot encounters an obstacle, the system identifies the type of object, then estimates its velocity and predicts its trajectory, calculates the most efficient path, and automatically performs an obstacle avoidance maneuver, rerouting the robot.
This navigation mode can boost efficiency, in particular in scenarios when robots share the shop floor with other robots, but it may not be the optimal solution when robots share the shop floor with people.
On the one hand, as human behavior is less predictable, robots have less visibility of a person’s movement intent than they have on other mobile robots or RTLS-tracked manned vehicles. On the other hand, having a robot overtake people while walking across a facility may be perceived as unpredictable and unsafe, making human workers feel anxious.
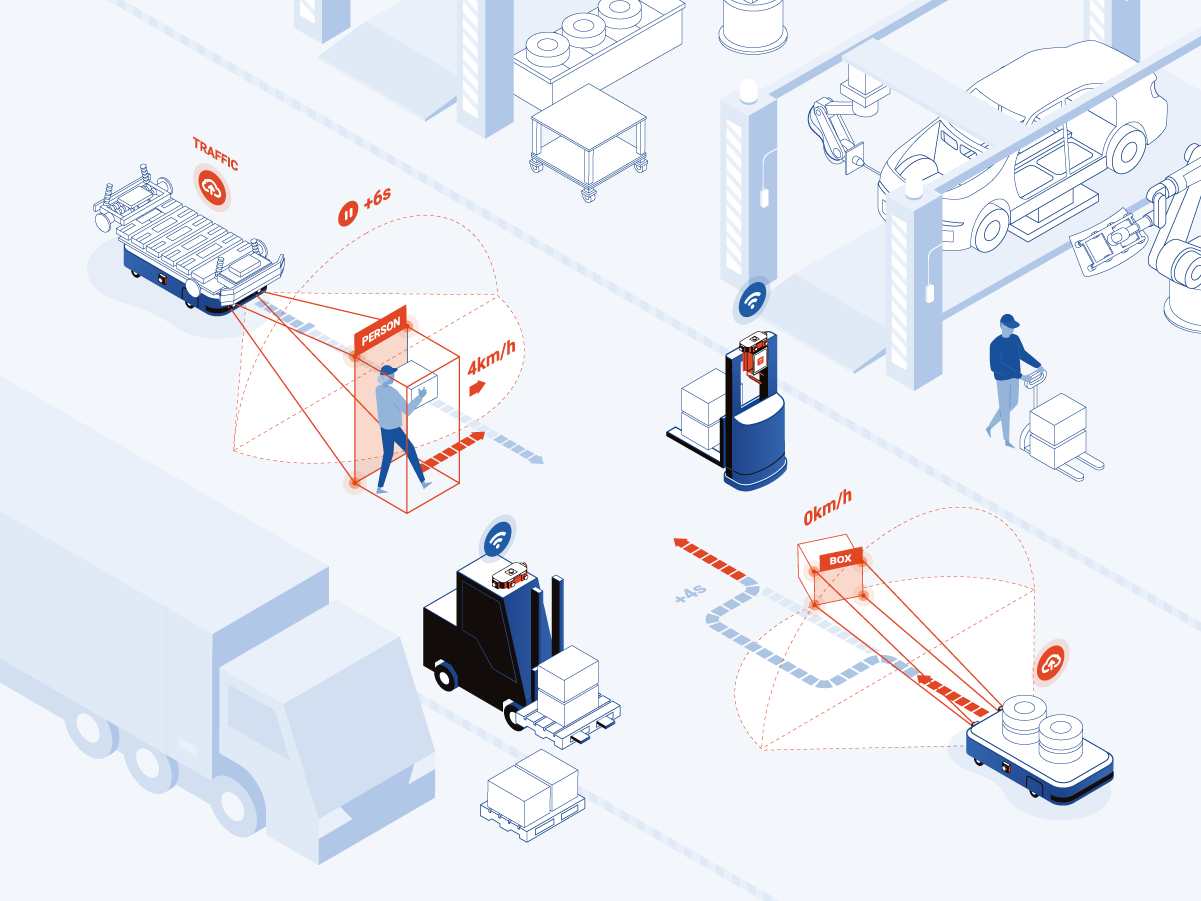
Solving the human-robot collaboration challenge is key to enabling Industry 5.0 and is motivating Sevensense’s work to solve this issue by combining scene understanding and a people-centric approach, to turn AMRs into Co-AMRs. The solution involves Visual AI perceiving the obstacle as a person and then commanding the robot to perform a people-friendly behavior such as stopping and waiting for the person to continue their movement or complete their task.
Looking into tackling the challenges of orchestrating mixed fleets, human-robot collaboration, or scaling operations? Get in touch!